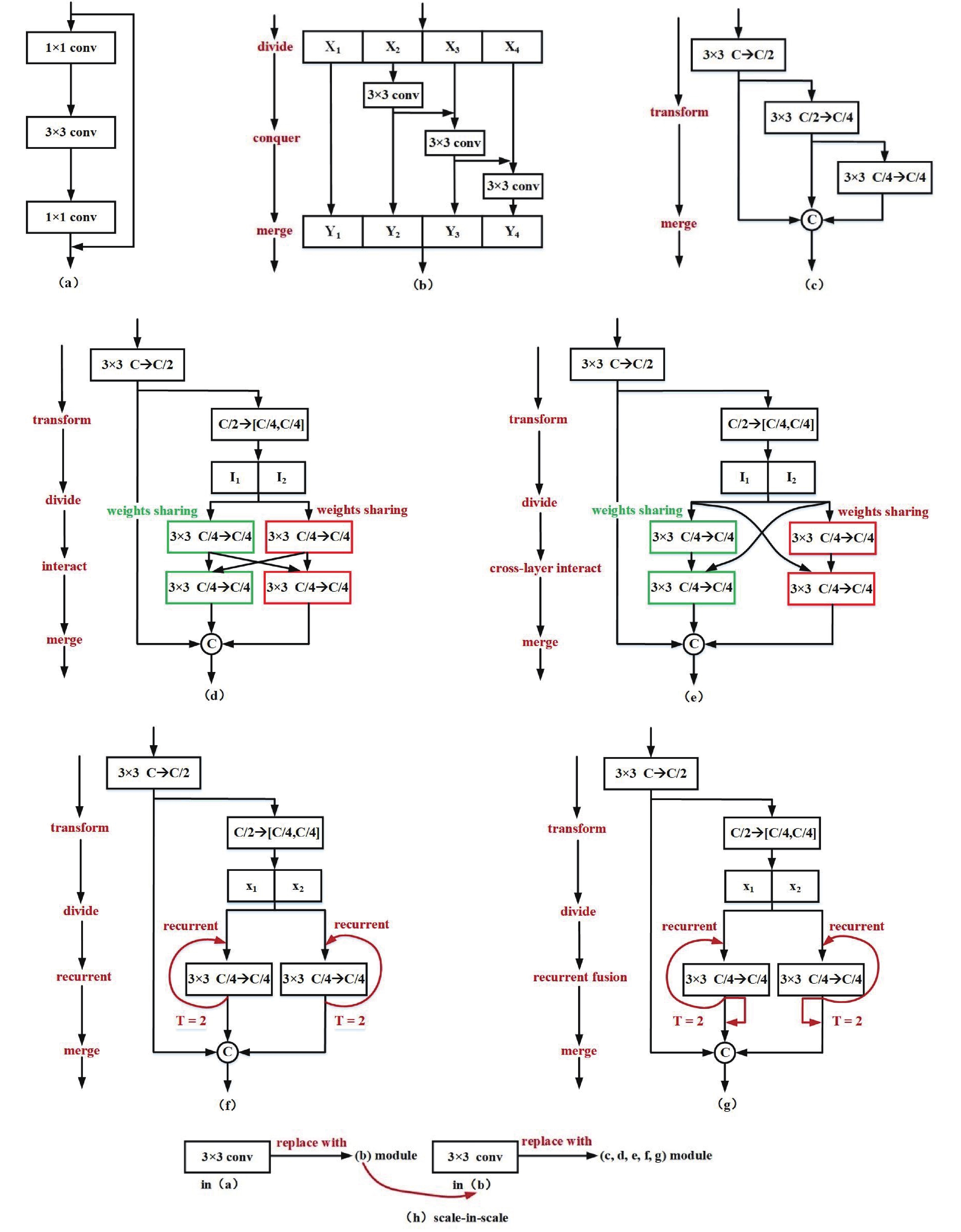
Visual features with high potential for generalization are critical for computer vision applications. In addition to the computational overhead associated with layer-by-layer feature stacking to produce multi-scale feature maps, existing approaches also incur high computational costs. To address this issue, we present a compact and efficient scale-in-scale convolution operator called SIS by incorporating an efficient progressive multi-scale architecture into a standard convolution operator. More precisely, the suggested operator uses the channel transform-divide-and-conquer technique to optimize conventional channel-wise computing, thereby lowering the computational cost while simultaneously expanding the receptive fields within a single convolution layer. Moreover, the proposed SIS operator incorporates weight-sharing with split-and-interact and recur-and-fuse mechanisms for enhanced variant design. The suggested SIS series is easily pluggable into any promising convolutional backbone, such as the well-known ResNet and Res2Net. Furthermore, we incorporated the proposed SIS operator series into 29-layer, 50-layer, and 101-layer ResNet as well as Res2Net variants and evaluated these modified models on the widely used CIFAR, PASCAL VOC, and COCO2017 benchmark datasets, where they consistently outperformed state-of-the-art models on a variety of major vision tasks, including image classification, key point estimation, semantic segmentation, and object detection.
We design a series of transformation mechanism to expand the module (a) and Res2Net module (b) into multi-scale module operators (c, d, e, f, g).
[1] |
Wang Q, Chen W, Wu X, et al. Detail preserving multi-scale exposure fusion. In: 2018 25th IEEE International Conference on Image Processing (ICIP). Athens, Greece: IEEE, 2018: 1713-1717.
|
[2] |
Wang B, Lei Y, Li N, et al. Multi-scale convolutional attention network for predicting remaining useful life of machinery. IEEE Transactions on Industrial Electronics, 2021, 68 (8): 7496–7504. DOI: 10.1109/TIE.2020.3003649
|
[3] |
Yu J, Xie H, Xie G, et al. Multi-scale densely U-Nets refine network for face alignment. In: 2019 IEEE International Conference on Multimedia & Expo Workshops (ICMEW). Shanghai, China: IEEE, 2019: 691–694.
|
[4] |
Zhang X, Zhang W. Application of new multi-scale edge fusion algorithm in structural edge extraction of aluminum foam. IEEE Access, 2020, 8: 15502–15517. DOI: 10.1109/ACCESS.2019.2963454
|
[5] |
Liu G, Wang C, Hu Y. RPN with the attention-based multi-scale method and the adaptive non-maximum suppression for billboard detection. In: 4th International Conference on Computer and Communications. Chengdu, China: IEEE, 2018: 2018.8780907.
|
[6] |
Sun K, Xiao B, Liu D, et al. Deep high-resolution representation learning for human pose estimation. In: 2019 IEEE/CVF Conference on Computer Vision and Pattern Recognition (CVPR). Long Beach, CA: IEEE, 2019: 5686–5696.
|
[7] |
Fang X, Yan P. Multi-organ segmentation over partially labeled datasets with multi-scale feature abstraction. IEEE Transactions on Medical Imaging, 2020, 39 (11): 3619–3629. DOI: 10.1109/TMI.2020.3001036
|
[8] |
Huang G, Chen D, Li T, et al. Multi-scale dense networks for resource efficient image classification. In: 6th International Conference on Learning Representations. Vancouver, Canada: ICLR, 2018.
|
[9] |
Moukari M, Picard S, Simon L, et al. Deep multi-scale architectures for monocular depth estimation. In: 2018 25th IEEE International Conference on Image Processing. Athens, Greece: IEEE, 2018: 2940–2944.
|
[10] |
Papyan V, Elad M. Multi-scale patch-based image restoration. IEEE Transactions on Image Processing, 2016, 25 (1): 249–261. DOI: 10.1109/TIP.2015.2499698
|
[11] |
Li J, Fang F, Li J, et al. MDCN: Multi-scale dense cross network for image super-resolution. IEEE Transactions on Circuits and Systems for Video Technology, 2021, 31 (7): 2547–2561. DOI: 10.1109/TCSVT.2020.3027732
|
[12] |
Lowe D G. Distinctive image features from scale-invariant keypoints. International Journal of Computer Vision, 2004, 60 (2): 91–110. DOI: 10.1023/B:VISI.0000029664.99615.94
|
[13] |
Szegedy C, Liu W, Jia Y, et al. Going deeper with convolutions. In: 2015 IEEE Conference on Computer Vision and Pattern Recognition (CVPR). Boston, MA: IEEE, 2015: 1–9.
|
[14] |
Gao S, Cheng M. M, Zhao K, et al. Res2Net: A new multi-scale backbone architecture. IEEE Transactions on Pattern Analysis and Machine Intelligence, 2019, 43 (2): 652–662. DOI: 10.1109/TPAMI.2019.2938758
|
[15] |
Krizhevsky A, Sutskever I, Hinton G. E. ImageNet classification with deep convolutional neural networks. In: Proceedings of the 25th International Conference on Neural Information Processing Systems: Volume 1. Red Hook, NY: Curran Associates Inc, 2012: 1097–1105.
|
[16] |
Simonyan K, Zisserman A. Very deep convolutional networks for large-scale image recognition. https://arxiv.org/abs/1409.1556.
|
[17] |
He K, Zhang X, Ren S, et al. Deep residual learning for image recognition. In: 2016 IEEE Conference on Computer Vision and Pattern Recognition (CVPR). Las Vegas, NV: IEEE, 2016: 770-778.
|
[18] |
Xie S, Girshick R, Dollár P, et al. Aggregated residual transformations for deep neural networks. In: 2017 IEEE Conference on Computer Vision and Pattern Recognition (CVPR). Honolulu, HI: IEEE, 2017: 5987–5995.
|
[19] |
Huang G, Liu Z, Van Der Maaten L, et al. Densely connected convolutional networks. In: 2017 IEEE Conference on Computer Vision and Pattern Recognition (CVPR). Honolulu, HI: IEEE, 2017: 2261–2269.
|
[20] |
Iandola F N, Han S, Moskewicz M W, et al. SqueezeNet: AlexNet-level accuracy with 50x fewer parameters and <0.5 MB model size. In: 5th International Conference on Learning Representations. Toulon, France: ICLR, 2017.
|
[21] |
Howard A G, Zhu M, Chen B, et al. Mobilenets: Efficient convolutional neural networks for mobile vision applications. https://arxiv.org/abs/1704.04861.
|
[22] |
Zhang X, Zhou X, Lin M, et al. ShuffleNet: An extremely efficient convolutional neural network for mobile devices. In: 2018 IEEE/CVF Conference on Computer Vision and Pattern Recognition. Salt Lake City, UT: IEEE, 2018: 6848–6856.
|
[23] |
Lin M, Chen Q, Yan S. Network in network. In: ICLR 2014 Conference.Banff, Canada: ICLR, 2014.
|
[24] |
Sun S, Pang J, Shi J, et al. FishNet: A versatile backbone for image, region, and pixel level prediction. Advances in Neural Information Processing Systems 31 (NeurIPS 2018). Montréal, Canada: NeurIPS, 2018: 754–764.
|
[25] |
Yu F, Wang D, Shelhamer E, et al. Deep layer aggregation. In: 2018 IEEE/CVF Conference on Computer Vision and Pattern Recognition. Salt Lake City, UT: IEEE, 2018: 2403–2412.
|
[26] |
Liao R, Zhao Z, Urtasun R, et al. LanczosNet: Multi-scale deep graph convolutional networks. In: Seventh International Conference on Learning Representations. New Orleans, LA: ICLR, 2019.
|
[27] |
Liu Y, Wang Y, Wang S, et al. CBNet: A novel composite backbone network architecture for object detection. Proceedings of the AAAI Conference on Artificial Intelligence, 2020, 34 (7): 11653–11660. DOI: 10.1609/aaai.v34i07.6834
|
[28] |
Chen L, Zhu Y, Papandreou G, et al. Encoder-decoder with atrous separable convolution for semantic image segmentation. In: Computer Vision–ECCV 2018. Cham, Switzerland: Springer, 2018: 833-851.
|
[29] |
Xiao B, Wu H, Wei Y. Simple baselines for human pose estimation and tracking. In: Computer Vision–ECCV 2018. Cham, Switzerland: Springer, 2018: 472–487
|
Models | Params | flops | Top-1 error |
ResNet-29 | 36.5M | 4.2G | 20.50 |
ResNext-29 | 34.4M | 4.2G | 17.90 |
Res2NeXt-29 | 33.8M | 4.2G | 16.93 |
Interactive SIS-I variant | 26.3M | 4.36G | 16.82 |
Interactive SIS-II variant | 26.5M | 4.36G | 16.79 |
Recurrent SIS-I variant | 25.7M | 4.52G | 16.87 |
Recurrent SIS-II variant | 25.9M | 4.52G | 16.85 |
Basic SIS variant | 28.3M | 4.2G | 16.74 |
Models | T=2 | T=3 | T=4 |
ResNet-29 | 20.50 | 20.45 | 20.38 |
ResNext-29 | 17.90 | 17.74 | 17.68 |
Backbone version | Basic SIS | Interactive SIS | Recurrent SIS | COCO | VOC07 | |||
I | II | I | II | AP | AP.5 | AP | ||
ResNet-50 | 29.8 | 49.7 | 71.5 | |||||
Res2Net-50 | 31.2 | 51.4 | 73.3 | |||||
SIS-50 variant | ✔ | 31.2 | 51.4 | 73.3 | ||||
✔ | 31.2 | 51.4 | 73.4 | |||||
✔ | 31.4 | 51.6 | 73.6 | |||||
✔ | 31.5 | 51.7 | 73.7 | |||||
✔ | 32.4 | 52.7 | 74.1 |
Backbone version | Basic SIS | Interactive SIS | Recurrent SIS | Mean IoU | |||
I | II | I | II | 50 variant | 101 variant | ||
ResNet | 0.751 | 0.770 | |||||
Res2Net | 0.767 | 0.781 | |||||
SIS variant | ✔ | 0.769 | 0.773 | ||||
✔ | 0.770 | 0.775 | |||||
✔ | 0.772 | 0.785 | |||||
✔ | 0.773 | 0.786 | |||||
✔ | 0.775 | 0.791 |
Backbone version | Basic SIS | Interactive SIS | Recurrent SIS | AR.5 | AR.75 | AR(M) | AR(L) | AR | AP(M) | AP(L) | AP.5 | AP.75 | AP | ||
I | II | I | II | ||||||||||||
ResNet-50 | 0.711 | 0.915 | 0.784 | 0.685 | 0.753 | 0.743 | 0.923 | 0.806 | 0.711 | 0.792 | |||||
Res2Net-50 | 0.721 | 0.916 | 0.802 | 0.696 | 0.761 | 0.752 | 0.929 | 0.821 | 0.723 | 0.797 | |||||
SIS-50 variant | ✔ | 0.723 | 0.916 | 0.803 | 0.697 | 0.765 | 0.754 | 0.929 | 0.823 | 0.725 | 0.800 | ||||
✔ | 0.724 | 0.917 | 0.804 | 0.698 | 0.767 | 0.756 | 0.929 | 0.824 | 0.726 | 0.801 | |||||
✔ | 0.726 | 0.916 | 0.805 | 0.701 | 0.767 | 0.757 | 0.929 | 0.827 | 0.727 | 0.803 | |||||
✔ | 0.727 | 0.925 | 0.804 | 0.700 | 0.767 | 0.758 | 0.931 | 0.825 | 0.726 | 0.805 | |||||
✔ | 0.731 | 0.925 | 0.814 | 0.706 | 0.772 | 0.761 | 0.932 | 0.831 | 0.731 | 0.808 | |||||
Res2Net-101 | 0.722 | 0.919 | 0.794 | 0.700 | 0.754 | 0.760 | 0.931 | 0.828 | 0.733 | 0.803 | |||||
SIS-101 variant | ✔ | 0.733 | 0.923 | 0.815 | 0.709 | 0.776 | 0.764 | 0.932 | 0.834 | 0.735 | 0.811 | ||||
✔ | 0.735 | 0.924 | 0.817 | 0.715 | 0.779 | 0.767 | 0.933 | 0.836 | 0.736 | 0.813 | |||||
✔ | 0.737 | 0.924 | 0.818 | 0.715 | 0.785 | 0.769 | 0.934 | 0.838 | 0.738 | 0.815 | |||||
✔ | 0.738 | 0.925 | 0.819 | 0.715 | 0.785 | 0.770 | 0.935 | 0.840 | 0.739 | 0.816 | |||||
✔ | 0.742 | 0.925 | 0.825 | 0.715 | 0.785 | 0.773 | 0.935 | 0.843 | 0.743 | 0.819 |